Primary sclerosing cholangitis (PSC) is one of the leading causes of liver transplantation in the Nordic countries. A chronic disease of the liver, PSC damages the bile ducts through inflammation and ultimately causes cirrhosis. As the disease progresses, the liver slowly loses its ability to function and, in some cases, can lead to bile duct cancer.
Nearly half of PSC patients do not exhibit symptoms and are only diagnosed by chance when abnormal liver function tests come back in an unrelated blood test. There are currently no effective treatments. The disease can even reoccur after liver transplantation, with some patients having to undergo several transplants. With the incidence of PSC increasing, it is imperative that those patients at risk of developing liver cirrhosis or cancer are identified early.
The problem
PSC is a complex and difficult disease. The pathogenesis is unclear; several theories exist, but they still need to be fully elucidated and confirmed. Diagnosis also proves tricky. This is due to the fact that PSC is versatile and heterogeneous. A patient sample can sometimes present normal in histopathological analysis despite the presence of disease. The biomarkers of interest in patient samples are also difficult to identify and quantify, while current methods rely upon manual analysis.
Nelli Sjöblom, an MD specializing as a pathologist at the Helsinki University Hospital in Finland, has studied liver samples for years. In 2018, she started a PhD project to identify prognostic markers for PSC that correlate with the progression of the disease and its development into cancer. Ultimately, Nelli’s aim is to help identify patients earlier, improve their outcomes, and formulate a scoring system for the disease.
Nelli came across a unique and information-rich set of PSC samples, part of a Helsinki patient registry founded by Professor Martti Färkkilä. From these samples of 318 patients, Nelli was able to classify the stages of their disease progression in cooperation with pathologist Sonja Boyd. Nelli and Sonja validated all the material to evaluate, from the patients’ liver biopsies, their disease’s stage. Nelli then wanted to delve in deeper to identify the histopathology that correlates with disease severity and possible development to cancer. However, when Nelli first started to formulate the scope of her PhD project, she knew she had to find a way to overcome the difficulties in analyzing liver samples in order to identify those key markers.
Automating the detection of key biomarkers
Nelli had been interested in artificial intelligence (AI) for a while and started to look for a way to incorporate it into her research. As Nelli explained: “A pathologist’s job really is image analysis, so AI felt like a natural tool to adopt due to its focus on this type of analysis.” However, as with most healthcare professionals, Nelli was pressed for time, not being able to commit to learning coding or how to use any complex and time-consuming software outside of her expertise.
A senior pathologist, Professor Johanna Arola, in her lab suggested Aiforia, as it would allow her to utilize deep learning AI to advance her PhD research without the need for any computing skills or burdensome software adoption. Nelli was, therefore, able to launch this unique project and, for the first time ever, automate the detection of key biomarkers in PSC.
Enabling research with AI
The first stage of deploying Aiforia for her research involved creating parameters in her samples. The AI model is taught these parameters when the user annotates and categorizes certain features in the image, such as liver tissue versus background. “Aiforia is built on a very user-friendly and intuitive interface. It took very little time to get started,” Nelli explains. With Aiforia and its capability for semantic segmentation, she was able to quickly train the AI model to see deeper and deeper — from liver tissue to bile ducts and hepatocytes.
Nelli was then able to start to analyze these samples for her first immunohistochemical marker of interest, CK7, the expression of which in the liver is commonly known as an indicator of bile duct injury. With samples stained for CK7, she could use Aiforia to create an algorithm to visualize and quantify the number of liver cells positive for this marker in her sample cohort of 318 patients.
“If a person started counting these cells one by one, they would lose their health and age.”
“And what’s more, the results are completely consistent. AI doesn’t have bad days like us humans do. Subjectivity is wiped away, while my work is significantly sped up. This is hugely important in pathology but also in research in general,” Nelli explains.
“A lot of quantitative data is being produced. This project would not be possible without AI,” said Nelli. She will soon analyze another biomarker with Aiforia and aims to then compare this data with the clinical information she has, hoping to reveal novel prognostic factors.
Aiforia enables researchers to access the quantitative data they need while allowing them to use their own expertise to make clinically significant conclusions and decisions. An AI model is not here to replace pathologists but rather to assist them, enabling more meaningful work.
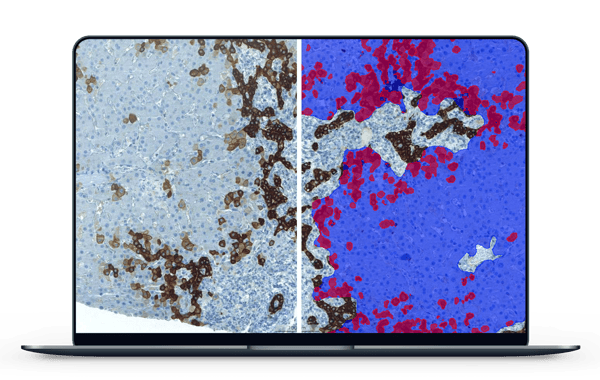
Conclusion
PSC is currently a very significant area of research globally. Many investigators and labs are working hard to identify prognostic markers, improve disease surveillance efforts, and ultimately improve patient outcomes. Nelli’s project is the first of its kind to harness the power of AI to identify the histopathology of PSC.
“When studying and determining prognostic indicators, it is vital that the data we are working with is reliable and objective — using AI, I have this trust in my data,” Nelli summarizes.
Aiforia not only enables the production of reliable data, but it also makes this easy, as Nelli’s case demonstrates. Deep-learning AI has the power to unlock the analysis and identification of crucial markers of disease, enabling new discoveries.
Read more case studies: