Challenges in breast cancer diagnostics
Rising rates of diseases like cancer have increased pathology sample numbers by 40% in the last 10 years (1). This has placed a significantly larger burden on pathologists, an already underrepresented group of healthcare professionals. Meanwhile, the tools currently available to pathologists do not reflect the ever-rising demand of their field.
A pathologist's workload consists mainly of a time-consuming, manual, and error-prone task: analyzing slides under a microscope. Consequently, patients often wait for long periods to receive a prognosis and are at risk for receiving ineffective treatments based on results for diseases that have since progressed. Both pathologists and patients deserve reliable and efficient tools for clinical use.
Breast cancer biomarker scoring methods currently used are particularly prone to variability. For example, commonly used scoring schemes necessitate pathologists to extract quantitative parameters that are subjected to inter- and intraobserver variability. Unfortunately, this means that the analysis is more qualitative.
The need for objective and standardized tools is evident. Deep learning artificial intelligence (AI) was intended to perform these repetitive quantification tasks — wholly immune to bias and variability. Analyses with AI can be replicated consistently, no matter the sample type, size, or any other human condition impairing its performance.
The solution: AI-assisted data and decision support
AI can help pathologists and patients overcome these issues. By automating time-consuming manual tasks, AI can count cells and biomarkers or identify tissue areas in a fraction of the time that humans can, allowing pathologists to deliver timely and accurate prognoses. An AI model trained to find metastasized breast cancer tumors was able to detect 92.4% of the tumors, compared to the human pathologist average of 73.2% (2). This hyperprecision increases diagnostic accuracy and, therefore, improves patient outcomes.
In addition to its clinical benefits, AI can help reduce costs and even out workload distribution. Reducing diagnostic error and misdiagnosis while improving treatment accuracy with more detailed results will provide direct cost savings due to greater precision. With fewer manual, repetitive, and time-consuming tasks to perform, pathologists can focus their time and energy on rare or complex cases that require more expertise to analyze.
Read more about the benefits of using AI in clinical diagnostics: 5 reasons to use AI in clinical diagnostics
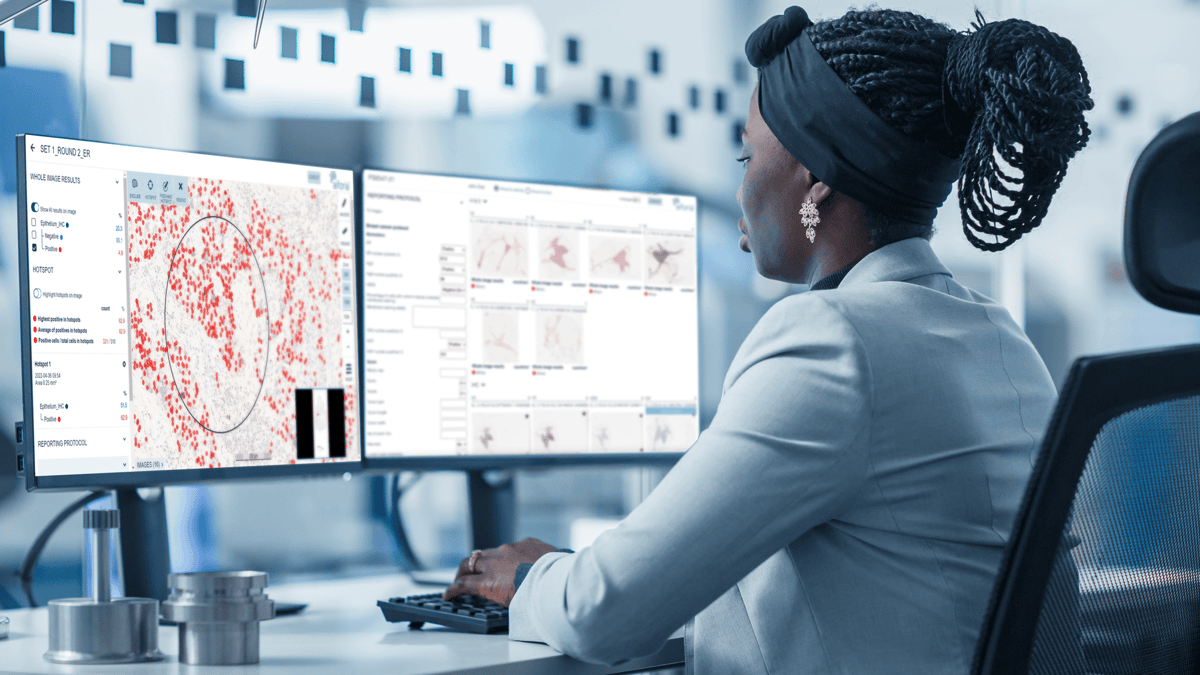
CE-IVD marked AI models for breast cancer
Aiforia currently offers three CE-IVD marked clinical AI models for breast cancer diagnostics as part of the Aiforia® Clinical Suite: clinical AI models for Breast Cancer: PR, ER, and Ki67. These models have the potential to improve the prognostic and predictive value of breast cancer diagnostics, helping pathologists to analyze medical images in a timely and consistent manner.
Aiforia® Breast Cancer PR automates the detection of tumor areas, the calculation of PR-positive and negative cells from whole slide images (WSIs) and selected areas, and the viewing and selection of areas with high PR-positive density or hotspots.
Aiforia® Breast Cancer ER automates the detection of tumor areas, the calculation of ER-positive and negative cells from WSIs and selected areas, and the viewing and selection of areas with high ER-positive hotspots.
Aiforia® Breast Cancer Ki67 automates the detection of tumor areas, the calculation of Ki67-positive and negative cells from WSIs and selected areas, and the viewing and selection of areas with high Ki67-positive hotspots.
Finally, the CE-IVD marked Aiforia® Clinical Suite Viewer provides pathologists with a cloud-based platform to view WSIs of human histopathological samples and deep learning AI-produced results, alongside their seamless reporting. Customizable to specific disease types, the Aiforia® Clinical Suite Viewer equips pathologists with the end-to-end solution they need to improve breast cancer diagnostics.
References
- Metter M, Colgan T, Leung, S, et al. Pathologists and Laboratory Medicine, 2019
- Liu Y, Gadepalli K, Norouzi M, et al. arXiv e-prints, 2017
