Aiforia® Create is the most versatile tool for developing deep learning AI models for image analysis. The intuitive user interface ensures fast annotation of training data, and no prior technical expertise is required to produce impactful applications.
Users can benefit from advanced image analysis features, including object detection, semantic segmentation, and instance segmentation. Let’s explore them in more detail below.
Object detection: identify any feature or pattern
Aiforia’s object detection technology can detect thousands of different objects in complex images. It enables multi-class identification (e.g., different types of immune cells) and the detection of rare objects (e.g., malaria-infected red blood cells) and can be combined with segmentation and spatial metrics for enhanced analysis.
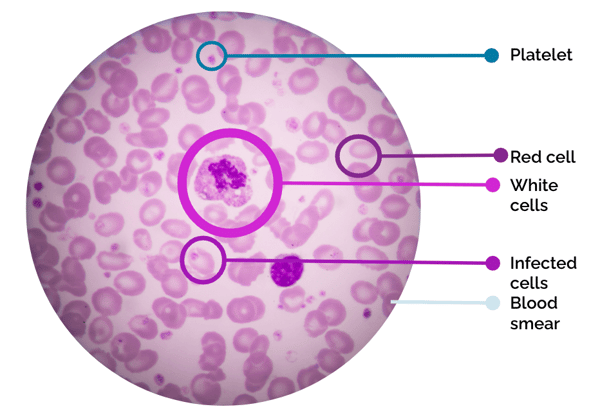
AI-supported object detection on blood smear
Semantic segmentation: enable area quantification
Semantic segmentation enables the detection of tissue, different classes of tumors, cells, and artifacts. It is typically the first layer of detection to find the area of interest.
Breast tissue epithelium/stromal segmentation and tumor grading serve as strong examples of applications for semantic segmentation.
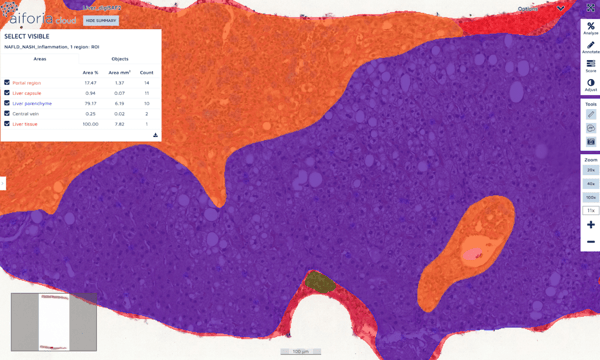
Multi-class segmentation of liver tissue sample
Instance segmentation: quantify merging or overlapping areas individually
AI-powered image analysis systems enable multiclass semantic segmentation and object detection, helping pathologists identify different region classes and object counts. However, calculating areas for segmentations and detected objects is challenging, especially with overlapping features, as seen in neuroscience.
Aiforia’s AI experts have addressed this with instance segmentation, an advanced technique that enables precise quantification of areas and shapes, even individually when areas merge or overlap. Image analysis can be taken a step further with instance segmentation as detailed data on aspects such, as area count, bounding, and staining intensity can be acquired.
.png?width=600&height=329&name=unnamed%20(1).png)
Identifying the full microglia and its individual processes with instance segmentation
Semantic segmentation vs. instance segmentation: what is the difference?
Semantic segmentation provides a holistic overview of the image by segmenting it into areas where individual objects may overlap. Instance segmentation enables more precise quantification of areas and shapes, even individually, when areas merge or overlap, allowing a more detailed analysis of true cell borders, their size, and dimensions.
Case examples of using instance segmentation
Showing the full microglia, in addition to the individual processes, despite some overlapping occurring in the image:
Detecting and quantifying infected cells in malaria:
Analyzing cancer biomarkers like Ki-67:
Want to see Aiforia’s AI in action? We can demonstrate the features on your images or any of Aiforia’s application examples. Request a free demonstration →
Get to know other Aiforia Create features: