Mycobacterium tuberculosis, the pathogen causing tuberculosis (TB), is a cunning species of bacteria. It can persist in hosts for years, evading their immune responses through many mechanisms. One such tactic is deregulating lipid metabolism, resulting in the formation of foamy macrophages. Therefore, these altered immune cells are a significant indicator of the presence of TB in a patient.
Traditional methods are manual and time-consuming
Gillian Beamer, a pathologist and assistant professor at Tufts University, has been studying experimental tuberculosis for 15 years. “Part of my work as a scientist and pathologist is to rank, score, grade, count, or otherwise quantify visual features. Typically, this means evaluation of tissue sections using a microscope and identification of differences amongst treatment groups,” Gillian describes.
This work is manual and very time-consuming. “For 15 years, I have been looking to train a computer to automatically recognize and count visual information,” Gillian explains. She found companies that would digitize slides and provide annotation tools, but taking it beyond managing her slides to extract information automatically proved difficult. “I wanted automated tools that could quantify complex visual information, producing quantifiable data instead of categorical or descriptive results,” she adds.
Speeding up the research with AI
After attending a digital pathology conference in 2018 and seeing a presentation from Aiforia, Gillian found the long-searched solution. “As soon as I saw Aiforia present, I knew that is what I needed!” she exclaims.
“I wanted a way to identify and quantify cell types and tissue changes efficiently.” However, many cell types and changes are straightforward to recognize. As Gillian explains it:
“They can be difficult to accurately quantify efficiently. I timed myself on a single slide once. I manually annotated every lesion type of interest, and it took 12 hours for one slide. This is not feasible for hundreds of slides and is mind-numbing. As a scientist and an experimental pathologist, what I want is the data. I do not want to be a ‘dot counter.”
Aiforia was deployed to create deep learning AI models to recognize and quantify foamy macrophage areas and count the cells within these identified spaces. Only 25 slides were needed for training with Aiforia® Create, Aiforia's AI development tool. “The time and effort to train the AI models was much less and more fun than I thought. The accuracy was higher, and the error rate was lower than I thought they would be.” Gillian describes.
Using Aiforia Create, she quickly became familiar with how deep learning works: “You get instant feedback on what it is recognizing and what it is not recognizing. Then, it is straightforward to make corrections. The iterative process is like working with a person, except, of course, the AI is much faster than 12 hours per slide and is never bored or tired!”
Benefits beyond the results
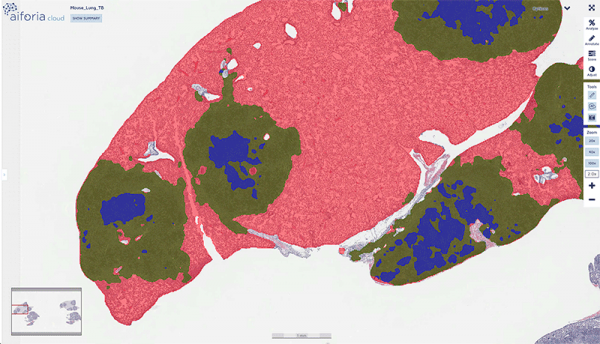
Samples from over 400 lungs of M. tuberculosis-infected mice were then scanned and analyzed with Aiforia Create. Gillian was impressed with the tool: “As well as rigorous quantification, which was my main interest, I can see that AI tools may better detect infrequent lesions/cells than human pathologists. The visual presentation of results is also great.”
Perhaps most importantly, though, the time-consuming counting is drastically reduced, as Gillian explains: “The pathologist now has time to analyze, generate new hypotheses, and think about results in the context of disease.” It took 15 seconds to analyze each slide for Aiforia Create, while it can take up to 12 hours for a trained and highly skilled pathologist to analyze that same slide. It is perhaps time to reconsider who does these manual tasks and who focuses on the bigger picture.
Read more case studies on infectious diseases: